Generative AI: What is it? Fundamentally, it is a technology that creates rather than merely analyzes. Imagine a group of extremely intelligent bees, each trained on a galaxy of data points, buzzing together to create software, images, and poems in response to your prompt. This is a creative renaissance driven by machine learning, not merely an evolutionary step. Generative AI systems can create completely new content based on learned patterns, in contrast to older AI models that only sorted, labeled, or predicted. The results are unique, frequently captivating, and sometimes indistinguishable from human work.
The concept of artificial creativity has gained popularity, especially since the advent of programs like ChatGPT, DALL·E, and Midjourney. A painting that has never been seen before appears when you type in a phrase, such as “a neon-lit fox flying over Tokyo.” Similar to this, you could request a Robert Frost-style poem or some whale-song-inspired ambient music, and the algorithm would provide it in a matter of seconds.
Generative AI Overview Table
Attribute | Detail |
---|---|
Full Concept Name | Generative Artificial Intelligence |
Notable Tools | ChatGPT, Gemini, Claude, DALL·E, Stable Diffusion, Midjourney, Sora |
First Major Breakthroughs | Variational Autoencoders (2013), GANs (2014), Transformers (2017) |
Key Players | OpenAI, Google DeepMind, Meta, Anthropic, IBM, Microsoft |
Real-World Applications | Creative writing, visual art, voice synthesis, drug discovery, customer support, code generation |
Risks and Challenges | Deepfakes, misinformation, bias, job displacement, copyright issues |
Prominent Use Cases | AI-written books, synthetic voices in video games, digital fashion design, AI in advertising |
Industry Trend | Compact domain-specific models outperforming larger generalist models |
Source for Reference | IBM Research on Generative AI |
Modern generative models are now able to interpret language, images, and sound with remarkable nuance thanks to the use of transformer architectures, which were first introduced by Google’s research paper Attention Is All You Need. These models analyze large amounts of text and pick up on minute variations in sentence structure, word choice, and tone. In stark contrast to the slower, sequential models of the past, this parallel processing enables much faster training and output.
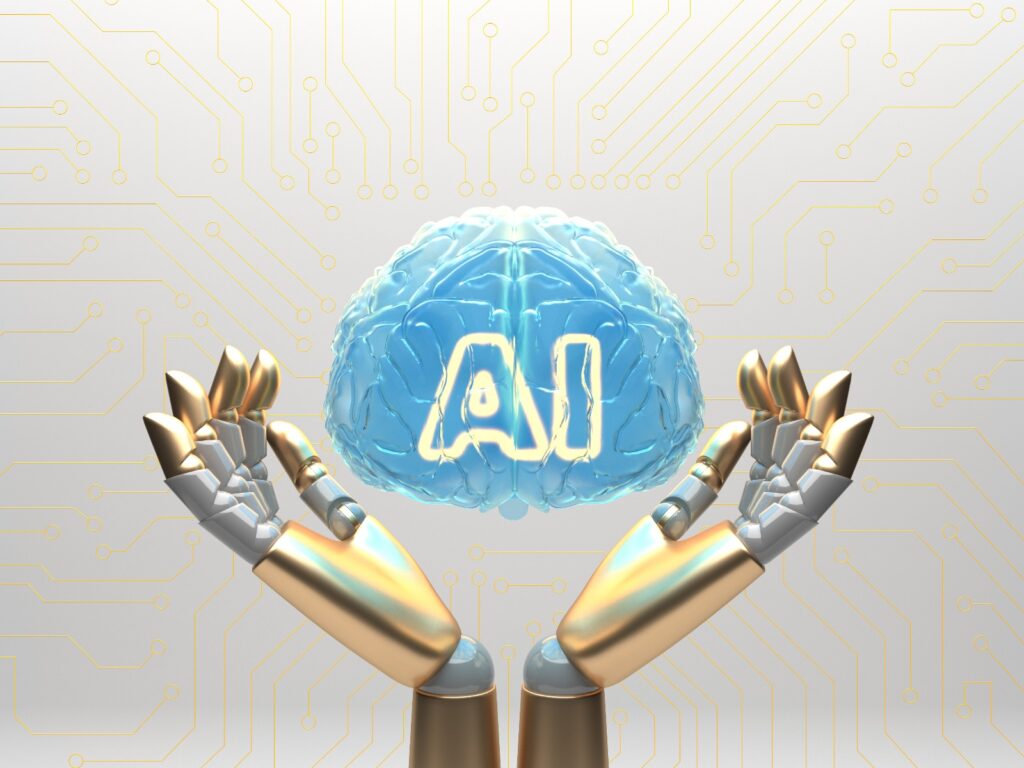
This change is best illustrated by the GPT series. With the introduction of an astounding 175 billion parameters, GPT-3 was able to mimic Shakespeare, write articles, and respond to philosophical queries. GPT-4 went one step further, improving the model’s ability to reason, decipher subtleties, and accurately follow intricate instructions. Simultaneously, Meta’s ImageBind, a highly adaptable tool for multimedia design and synthetic reality applications, blurred the lines between media formats by training models to comprehend images, audio, and even temperature data simultaneously.
These capabilities have already been adopted by marketers, developers, and artists. In a public statement, singer Grimes offered to split royalties with fans in exchange for her voice in AI remixes. A cultural divide between creators who see AI as a collaborator and those who see it as an imposter is revealed when Nick Cave called AI-generated songs that mimic his style a “grotesque insult.”
However, its uses are not limited to the arts. Generative models have started creating new pharmaceutical compounds by using historical chemical data as training data, which has drastically shortened research timelines. These models create artificial datasets in the financial services industry to enhance fraud detection while protecting consumer privacy. AI-generated agents now react in customer service interactions in a tone that is so natural that many users are unable to tell them apart from human operators.
There are urgent issues raised by this quick evolution as well. Misinformation has been fueled by the use of deepfake videos and artificial voices to mimic celebrities and politicians. Artists have complained that their creations are being uninvitedly scraped into training data. In response, scholars and policymakers are investigating methods for creating transparent licensing models and watermarking AI-generated content. The goal of these initiatives is to balance intellectual property protection with innovation.
There has been a discernible shift in favor of specialized models in recent months. Instead of training large general-purpose engines, scientists are creating small models that are optimized for particular industries. On clinical questions, PubMedGPT, which was trained on medical literature, has performed noticeably better than larger models. This change implies that smaller models that are adapted to specific data sources might be more effective and reliable when domain accuracy is important.
The way open-source communities are democratizing access to generative AI is equally compelling. By creating training data from the larger system itself, Stanford’s Alpaca, for instance, was able to condense the capabilities of GPT-3.5 into a smaller, faster model. This technique successfully teaches a smaller model to mimic a giant. Numerous spin-offs, such as Vicuna and Dolly, have been sparked by this method, indicating a larger trend: AI’s potential is no longer constrained by billion-dollar barriers.
China, meanwhile, has quickly become a leader in generative AI. More than 80% of Chinese professionals now use generative AI, compared to 65% in the United States, according to SAS and Coleman Parkes Research. The nation’s aggressive investment strategy is demonstrated by the more than 38,000 GenAI patents it filed between 2014 and 2023. The EU, on the other hand, is putting safety and regulation first and creating frameworks that try to maintain ethical considerations at the forefront.
Businesses like IBM are creating especially creative methods to incorporate generative AI into enterprise systems through targeted research and strategic alliances. The options are both realistic and motivating, ranging from using AI-generated synthetic data for model training to writing reliable software code with tools like Watsonx.
Reinforcement learning from human feedback (RLHF) and instruction tuning have become crucial in directing model behavior as capabilities grow. By tailoring the AI’s responses to human preferences, these techniques increase the relevance and emotional resonance of the outputs. RLHF transforms raw intelligence into a friendly, useful interaction by polishing the conversation and matching user intent.
It is anticipated that these tools will be integrated into everyday processes in the upcoming years. Imagine a fashion designer explaining a collection or an architect verbally sketching out building concepts, then watching as real-time visuals develop in response to their commands. Generative AI is poised to evolve from a tool to a digital creative partner by incorporating user prompts and precisely adjusting outputs.
Even with the brilliant potential, prudence is still required. Sometimes, generative models are prone to “hallucinations”—providing certain answers that are incorrect in terms of facts. If left unchecked, these errors could erode public confidence in AI applications. However, researchers are creating systems that are more grounded, ethically guided, and aware of context thanks to advancements in alignment techniques, transparency tools, and parameter-efficient tuning.